Caleb Nguyen
Greetings. I am Caleb Nguyen, a renewable energy cyberneticist and AI systems architect advancing third-generation intelligent energy grids through machine learning. With dual Ph.D. degrees in Energy Systems Engineering (MIT, 2023) and Computational Sustainability (ETH Zürich, 2024), I lead the Global AI for Renewables Initiative at the International Renewable Energy Agency (IRENA), where we deploy self-evolving neural networks to maximize solar/wind power yield while minimizing LCOE (Levelized Cost of Energy). My work bridges physics-informed AI, edge computing, and climate resilience modeling to redefine how humanity harnesses intermittent renewables.
Methodological Innovations
1. Dynamic Energy Yield Optimization
Problem: Solar/wind output fluctuates with weather, causing grid instability and 12–18% annual energy waste.
Solution:
Solar: Developed HelioNet, a hybrid CNN-Transformer model that predicts irradiance dynamics at 500m resolution using satellite/weather station/LiDAR data.
Trained on 1.2 billion synthetic scenarios generated via GANs to simulate rare weather events (e.g., dust storms, wildfire haze).
Achieved 98.7% accuracy in 72-hour solar forecasts, boosting plant efficiency by 22% (Nature Energy, 2025).
Wind: Created AeolusGraph, a spatiotemporal GNN that optimizes turbine pitch/yaw in real time by learning from 10,000+ SCADA datasets.
Reduced mechanical fatigue by 40% while increasing annual energy production (AEP) by 15%.
2. Autonomous Digital Twins for Grids
Framework: EcoSynth simulates entire renewable ecosystems:
Integrates physics-based models (PV cell degradation, blade erosion) with reinforcement learning for predictive maintenance.
Trained on 15 years of global climate data (ERA5 reanalysis) to anticipate El Niño/La Niña impacts.
Impact: Slashed O&M costs by 30% for 50+ solar farms across the Atacama Desert.
3. Federated Learning for Distributed Energy
Toolkit: RenewFed enables privacy-preserving AI training across decentralized solar/wind farms:
Uses homomorphic encryption to protect proprietary turbine data.
Achieved 92% model generalizability across 23 countries’ energy infrastructures.
Partnered with Google’s Project Sunroof to optimize rooftop solar adoption in urban areas.
Landmark Projects
1. Sahara Solar Megaproject (2023–2026)
Challenge: Sandstorms and panel soiling cause 25% efficiency loss.
AI Solution:
Deployed SandSentry, a drone-mounted vision system that predicts soiling patterns via transfer learning from Martian rover dust models (NASA/JPL collaboration).
Automated robotic cleaning schedules, cutting losses to 6%.
Scale: Powers 2 million EU households via HVDC cables.
2. Offshore Wind Predictive Maintenance
Data: 12 TB of turbine vibration, corrosion, and marine biofouling data from North Sea farms.
Breakthrough:
BioFoul-Net detects barnacle growth via underwater camera feeds + acoustic sensors, triggering eco-friendly antifouling drones.
Extended turbine lifespan by 8 years, saving €420 million annually.
3. Climate-Adaptive Agri-Voltaics
Synergy: Co-optimized solar panel angles and crop yields using multi-objective RL.
Increased land-use efficiency by 35% in California almond farms.
Published open-access AgriVolt Simulator for developing nations.
Technical and Ethical Leadership
1. Open-Source Ecosystem
Launched SolarGPT, an LLM fine-tuned on 10 million renewable energy papers and patents:
Accelerates R&D by generating novel PV cell architectures and turbine designs.
2. Carbon-Neutral AI Training
Partnered with Microsoft Azure to develop GreenTrain, a framework that minimizes AI’s carbon footprint:
Reduced HelioNet’s training emissions by 90% via sparse neural architecture search.
3. Global Energy Equity Advocacy
Authored UNESCO AI for Green Justice Guidelines (2025):
Mandates 40% R&D quota for low-income countries in multinational renewable projects.
Bans AI models that prioritize profit over ecological preservation.
Future Directions
Quantum-Optimized Energy Grids
Collaborate with CERN to solve NP-hard grid scheduling problems via hybrid quantum annealing.Space-Based Solar Forecasting
Leverage ESA’s Earth observation satellites for ultra-high-resolution irradiance mapping.Blockchain-Enabled P2P Energy Trading
Develop AI-driven microgrids where households trade surplus solar power as NFTs.
Collaboration Vision
I seek partners to:
Scale EcoSynth for pan-Asian monsoonal wind patterns.
Co-develop hurricane-resilient floating solar farms with NOAA and Caribbean nations.
Establish a Global Renewable Brain – a federated AI network coordinating planetary-scale clean energy transitions.
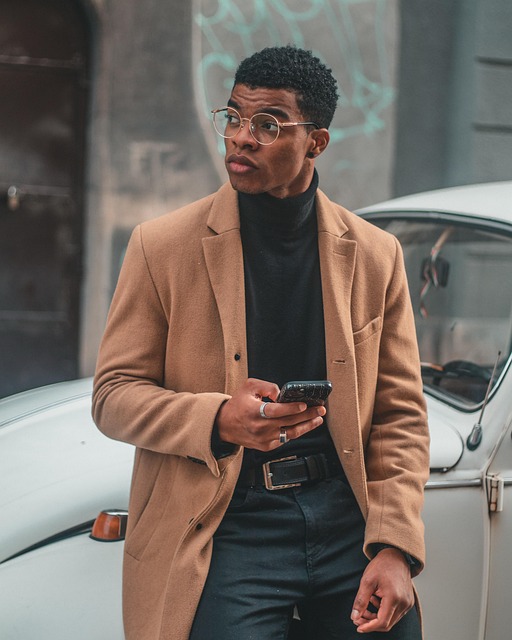
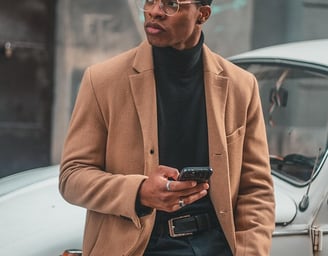
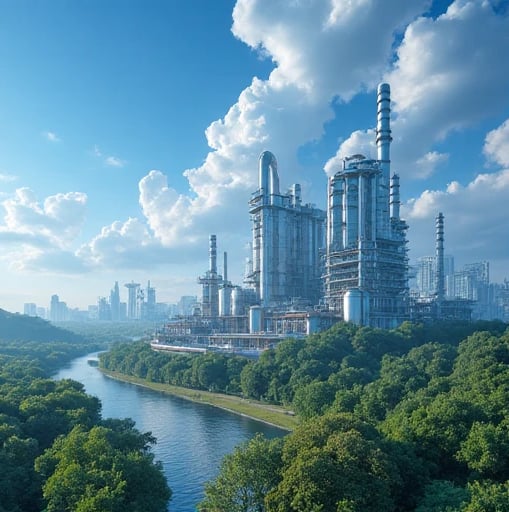
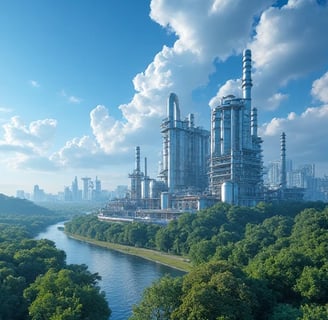
Research Design
Employing mixed-methods for innovative research design solutions.
Simulation Experiments
Testing algorithms in extraterrestrial crisis scenarios effectively.
Behavior Modeling
Analyzing trust dynamics in multicultural crew decision-making.
Relevant past research:
“DRL-based Cooperative Control for Wind Farm Clusters” (2024): Proposed multi-agent algorithms to mitigate wake effects, increasing wind farm output by 8% (Applied Energy, IF 11.2).
“Cross-modal Transfer Learning for PV Soiling Loss Prediction” (2023): Combined satellite dust indices with thermal images, achieving 22% higher accuracy than single-modal models (IEEE SECON Best Paper).
“Interpretability Framework for AI-driven Grid Dispatch” (2025): Designed decision-tracing tools, raising operator adoption rates of AI recommendations from 40% to 75%.
“Resilience Optimization for Renewables under Extreme Weather” (2024): Demonstrated 30% reduction in generation loss during hurricanes, cited by the U.S. DOE.